Northeast Region Workforce Vector Data
Type your county name in the dropdown menu and then select it from the list.
Workforce Vector data for your county will be displayed in the tables.
[See Note A below.]
Workforce Vector FAQs, definitions, and notes are included beneath the Workforce Vector tables.
These pages need to be viewed on a desktop computer.
The mobile-friendly version is still in development.
NOTE: This is PRELIMINARY data and is subject to change.
[See Note B below.]
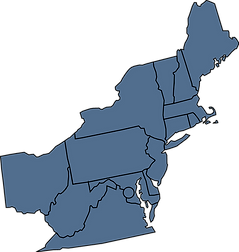
County:
General Information
0.1 Population
0.2 Population 16+
0.3 Civilian Labor Force 16+
County Total
County:
Employee Output/yr
1.1 Capital Investments
1.2 Labor Composition
1.3 Process Improvements
% GDP/yr Rank ENE/yr Workforce Potential/yr
County:
Retention/yr
% Emp./yr Rank Employed/yr Workforce Potential/yr
2.1 Quits and Discharges/year
2.2 Layoffs/year
2.3 Out-Migration Rate/year
Population
Population
Population
Population
Population
Population
Population
Population
County:
Not in Labor Force
% Not in LF Rank # Not in LF Workforce Potential
3.1 Students 14-15 [Warning! See notes.]
3.2 Non-Students 16-19
3.3 Students 16-19
3.4 Females 20-54
3.5 Males 20-54
3.6 Individuals 55-74
3.7 Females with Children < 6
3.8 Foreign Born - Naturalized Citizens
3.9 Foreign Born - Not a U.S. Citizen
3.10 Individuals with a Disability
3.11 Indiv. Experiencing Homelessness
3.12 Indiv. with Income < Poverty Level
3.14 Individuals Recently Incarcerated
3.15 Latinos
3.16 Part-time Employees
3.17 Unemployed [See notes.]
3.18 Veterans
3.19 Volunteers (FTE)
3.13 Indiv. without a College Degree
Population
Population
Population
Population
County:
Employed Elsewhere
% Base Rank Employed Workforce Potential
4.1 In-commuters
4.2 Out-commuters [Warning! See notes.]
4.3 Remote Workers
4.4 In-state Migration/year
4.5 Out-of-state Migration/year
4.6 International Migration/year
4.7 Refugees/year
4.8 Contract / Temp Workers
4.9 Part-time to Full-time Employees
4.10 Poaching/year
4.11 Second-job Employees
4.12 Self-employed Individuals
Population
Population
Population
Population
Population
Population
Population
Population
Population
Population
Population
Population
Population
Population
Population
Population
County:
Future Employees
% Base Rank Total Workforce Potential
5.1 Apprenticeships/year
5.2 Internships
5.3 Youth Programs
Population
Population
Population
Population
FAQs
How can I learn more about what these numbers mean?
There are a number of resources on the site to help explain the Workforce Vector and how it is used to help organizations grow their workforce.
-
The 40 workforce elements (the rows in the tables above) are described here.
-
The potential to increase employment of each type of individual is described here.
Can we talk about how this can help me find employees?
If you would like to have a brief conversation about how this data can be used to increase or retain your workforce, send me an email at ron.cox@midwestworkforce.com or contact me through my website contact form.
How accurate are these numbers?
CAUTION! The numbers provided here are approximations and should only be used to get a sense of local conditions and possibilities. The data should only be used to compare and contrast options and to guide the user as they explore more local and more timely information.
The data is not exact for the following reasons.
-
The Federal data are approximations based on surveys. All values have an error bound, meaning the result displayed is the best estimate but there is a chance the actual value is different, but within some error bound. For instance, a county estimate for a group size could be 120 with a margin of error of 75. The value 120 is the estimate of the group size (displayed in the table above) and the 75 is the margin of error. See www.bls.gov for more information on margins of error and confidence level. Error margins are not included in the table above. Note that the accuracy of the estimates tend to be better (lower margin of error as a percent of the estimate) for larger populations where the sampling size is larger. Estimates for low population counties tend to have much higher error ranges as a percent of the estimate.
-
Five year averages are given for the majority of the values in the table. This is done to reduce the short-term fluctuations in the data and because data is not released annually for small counties because of the small sample size.
-
Some data is not known at the county level, so statewide or national values for some variables are used in conjunction with local data (if available) to estimate local conditions. The errors in these estimates are greater when local conditions differ significantly from the state or national averages used in the calculations.
Definitions
(*): An asterisk indicates the workforce vector values shown (except for the potential) are an average of a portion of the counties in the state. This generally arises for small counties when Federal data is not available. See the FAQs (above) and the specific workforce element note (below).
ENE: Equivalent New Employees per year.
FTE: Full Time Equivalent.
Labor Force: Individuals employed and unemployed.
NA: Data not available.
NILF: Not In the Labor Force
% Not in the LF: The fraction of the civilian population 16+ that is not in the labor force. They are not employed nor are they unemployed.
Population: Table entries marked as 'Population' are currently being created and will be updated over the next several months.
RUCC: Rural-Urban Continuum Codes.
Workforce Potential: The number of additional individuals that might be able to be pulled into a county's labor force if productivity, hiring, and retention rankings are improved by 10% from the median county values.
Rank: The county place within all U.S. counties in terms of the percent employed. 1% = Top 1% (highest fraction employed or lowest fraction not in the LF); 100% = 99-100% (lowest fraction employed or highest fraction not in the LF).
Workforce Element Notes
Alphabetical general notes are listed first. These are followed by notes specific to each workforce element. Additional information will be added to the notes in the coming months. Notes will change over time as research continues.
[A] The government combines some data areas so your ‘County’ name listed here may be slightly different. This applies to Alaska, Connecticut, and Virginia. Data for U.S. territories is not included in this work.
[B] This work is on-going research and will change over time. All of the numbers presented here have errors of varying magnitudes. These errors occur because of the statistical modeling used by those creating the data, by changes in data over time, by the use of national or state-level data for county level information when data at the local level is not known, and by estimates made by the author when certain information is not known at the local level. See the FAQs above for more information.
Some estimates of workforce element data are questionable and extreme caution should be used. Typically this occurs when there are very large variations in a quantity and the definition of the term and the actual data gathered by BLS or the Census Bureau differs. Additional errors can occur when local data is not available and state or national averages are used to arrive at an order of magnitude for a term. An example is Out-commuting. See 4.2 below.
0.1 Average of 2018-2022 county data. Population 0+ years old.
0.2 Average of 2018-2022 county data. Population 16+ years old.
0.3 Average of 2018-2022 county data. Civilian employed and unemployed 16+.
1.1 Annual data. Least-square linear fit to 2013-2022 state productivity data. State data is adjusted slightly to approximate county-level productivity changes. A limiter is applied to an annualized 2013-2023 GDP/hr county estimate to constrain the variation off of the state average. Annualized percent changes in county GDP/hr are split into capital, composition, and TFP components by assuming every county looks like the national average splits. The county estimate for capital may be higher or lower than the value shown because of the county mix of industry, labor, and other factors compared with the national and state averages of industry, labor, and other factors. The equivalent number of new employees is the percent GDP/hr change multiplied by the employment level (2018-2022 data). Base population = Employed 16+.
1.2 Annual data. Least-square linear fit to 2013-2022 state productivity data. State data is adjusted slightly to approximate county-level productivity changes. A limiter is applied to an annualized 2013-2023 GDP/hr county estimate to constrain the variation off of the state average. Annualized percent changes in county GDP/hr are split into capital, composition, and TFP components by assuming every county looks like the national average splits. The county estimate for capital may be higher or lower than the value shown because of the county mix of industry, labor, and other factors compared with the national and state averages of industry, labor, and other factors. The equivalent number of new employees is the percent GDP/hr change multiplied by the employment level (2018-2022 data). Base population = Employed 16+.
1.3 Annual data. Least-square linear fit to 2013-2022 state productivity data. State data is adjusted slightly to approximate county-level productivity changes. A limiter is applied to an annualized 2013-2023 GDP/hr county estimate to constrain the variation off of the state average. Annualized percent changes in county GDP/hr are split into capital, composition, and TFP components by assuming every county looks like the national average splits. The county estimate for capital may be higher or lower than the value shown because of the county mix of industry, labor, and other factors compared with the national and state averages of industry, labor, and other factors. The equivalent number of new employees is the percent GDP/hr change multiplied by the employment level (2018-2022 data). Base population = Employed 16+.
2.1 Annual data. On-going research.
2.2 Annual data. On-going research.
2.3 Annual data. Average of 2018-2022 county data. Fraction of the population 0+ that out-migrates each year. The number of out-migrators is adjusted lower by the identical amount in every county, by a national estimate, to approximate the number of employees that leave each county annually. This is on-going research and this number may be adjusted lower. Base population = population 0+.
3.1 The Students 14- to 15-years-old workforce element should be used with caution since there is very little research data available. The 14-15 population size is estimated from a 10-14 population value and a 15-17 value. The unemployment rate is assumed to be the same as the rate for the population 16-19, based on research prior to 2000. The employment participation rate is estimated to be a fraction of the 16-19 rate, based on research prior to 2000. There are many root causes associated with the 14-15 age group, which make it difficult for individuals to find employment. This group is much more likely than other groups to work part-time and part-year, which further limits the impact the group can have on the local labor force. Average of 2018-2022 county population data. Base population = Population 14-15 estimate.
3.2 Average of 2018-2022 county population data. Base population = Non-student population 16-19.
3.3 Average of 2018-2022 county population data. Base population = Student population 16-19.
3.4 Average of 2018-2022 county population data. Base population = Female population 20-54.
3.5 Average of 2018-2022 county population data. Base population = Male population 20-54.
3.6 Average of 2018-2022 county population data. Base population = Population 20-54.
3.7 Females 20-64 with children less than six years old. Average of 2018-2022 data. Base population = Civilian females 20-64.
3.8 Data is only available at the county level for large-population counties. This data, together with the state average, is used to compute an average value for the fraction of the foreign-born not in the labor force in the small-population counties. When error bounds lead to a large variation in the estimate for the small-county average, the NILF estimate is limited to 1.5 standard deviations (of all states) from the state average. An asterisk (*) is used to indicate when a small-county average is used. Small counties with industries that tend to hire more/fewer foreign-born individuals will likely have a greater/smaller value of those not in the labor force than indicated by the average. Average of 2018-2022 state and county population data. Base population = Foreign-born - naturalized citizen population 16+.
3.9 Data is only available at the county level for large-population counties. This data, together with the state average, is used to compute an average value for the fraction of the foreign-born not in the labor force in the small-population counties. When error bounds lead to a large variation in the estimate for the small-county average, the NILF estimate is limited to 1.5 standard deviations (of all states) from the state average. An asterisk (*) is used to indicate when a small-county average is used. Small counties with industries that tend to hire more/fewer foreign-born individuals will likely have a greater/smaller value of those not in the labor force than indicated by the average. Average of 2018-2022 state and county population data. Base population = Foreign-born - not a U.S. citizen population 16+.
3.10 Average of 2018-2022 county data. Base population = Individuals with a disability 18-64.
3.11 This data is for the sheltered homeless, which is about one-half of all individuals experiencing homelessness. Some of the sheltered homeless are in the labor force, so the number NILF is less than the total in shelters. There is no data at the state or county level on the fraction of the sheltered homeless that are not in the labor force. The value provided here is an estimate based on 1) state data (2018-2022) on the fraction of the state population in shelters, 2) national data on the fraction of the sheltered homeless 16+ years old (assumed to hold in all counties), 3) the national value for those in shelters who are not in the labor force (assumed to hold in all counties), and county data on the population 16+ (2018-2022). The workforce potential is computed using a different approach because of the lack of NILF data. The potential is the increase in labor available if homelessness decreases in comparison to peers. The potential value is low in part because some of those in shelters are already employed. Since the fraction of individuals experiencing homelessness tends to be higher in urban areas, urban data is likely under-estimated and rural data is likely over-estimated. Base population = population 16+.
3.12 Individuals with income less than the poverty level. Average of 2018-2022 data. Base population = Civilian labor force 16+.
3.13 Individuals without a college degree only includes those without a high school degree and those with a high school degree that did not complete any higher education courses. Individuals who completed some college-level courses but did not get a degree are not included. Average of 2018-2022 county data. Base population = Individuals 25-64 with high school as the highest degree.
3.14 On-going research.
3.15 Average of 2018-2022 county population data. Base population = Latino civilian population 16+.
3.16 Average of 2018-2022 county data. The 'Not in Labor Force' value is all individuals 16+ not in the labor force. It is assumed that individuals entering the labor force will distribute more favorably to part-time employment. Specifically, to the average of a) completely part-time and b) the county ratio of part-time to full-time employment. Base population = Population 16+.
3.17 Average of 2018-2022 county data. The unemployed are technically considered to be in the labor force. The other workforce elements in this category highlight those that are not in the labor force. That is, individuals who are neither employed nor unemployed. The county unemployment rate is shown in the '% Not in LF' column. Base population = Civilian population 16+.
3.18 Average of 2018-2022 county data. Base population = Veterans 18-64.
3.19 Average of 2018-2022 county data on the population 16+. Volunteering rate is an average of 2017, 2019, and 2021 data. Volunteer data is only known at the state level so it is assumed that the county volunteer rate equals the state average. The non-volunteer rate is shown in the '% Not in LF' column. The '# Not in LF' and the Workforce Potential are converted to an equivalent, fully-employed person using the national average of the number of volunteer hours per volunteer.
4.1 On-going research.
4.2 The out-commuting workforce element is known to have large errors. Differences in remote work, hybrid work, and other work-location situations impact government survey results, so caution should be used when reviewing results. In addition, the ability to change commuting patterns can be difficult in certain situations. For instance, when a professional is commuting from a small county with poor job fit opportunities to a nearby metropolitan area. The ability to change this commuting behavior is less than what would be predicted by the perturbation methods used in this work to estimate potential changes. Average of 2018-2022 county population data. Base population = Employed 16+.
4.3 Average of 2018-2022 county data. Includes individuals who usually worked from home, so this includes some hybrid work. Remote work varies by industry, so variations in industry mix by county will affect the potential calculation. As such, the potential should be considered an upper estimate. Base population = Employed 16+.
4.4 Annual data. Average of 2018-2022 county data. The in-state migration rate is the number of individuals moving to the county per year from within the state, as a percent of the county population 1+. The in-state migration rate is a modified rate to adjust for states with (typically) one very large population county compared to the rest of the population in the state. The number of migrators is adjusted lower by an identical amount in every county, by a national estimate, to approximate the number of employees migrating. This is on-going research and this number may be adjusted lower. Base population = population 1+, adjusted for county population skew.
4.5 Annual data. Average of 2018-2022 county data. The in-migration rate is the number of individuals moving to the county from other states per year, as a percent of the county population 1+. The number of out-of-state migrators is adjusted lower by an identical amount in every county, by a national estimate, to approximate the number of employees migrating. This is on-going research and this number may be adjusted lower. Base population = population 1+.
4.6 Annual data. Average of 2018-2022 county data. The immigration rate is the number of individuals moving to the county from other countries per year, as a percent of the county population 1+. The number of immigrates is adjusted lower by an identical amount in every county, by a national estimate, to approximate the number of employees migrating. This is on-going research and this number may be adjusted lower. Base population = population 1+.
4.7 Annual data. Average of data from 2018-2022 for county population and from 2013-2023 for refugees/year. Assumes refugees are distributed proportional to the local population. When refugees arrive, they often are more concentrated in counties that have more Federal and local resources. See: https://www.acf.hhs.gov/orr/map/find-resources-and-contacts-your-state. Refugee estimates are likely over-estimated in counties with few resources and under-estimated in counties with more settlement resources. The number of refugees is adjusted lower by an identical (national) amount in each county to approximate the number of refugees that will be in the labor force. This is on-going research and this number may be adjusted lower. Base population = Population 0+.
4.8 On-going research.
4.9 Average of 2018-2022 county data. The potential is the conversion of current part-time employees to full-time employees, not new full-time employees from those not-employed. Base population = Full-time and Part-time/Part-year population 16+.
4.10 On-going research.
4.11 On-going research.
4.12 County estimates for the number of self-employed (SE) individuals with no employees are made by using 1) 2018-22 county data on the number of employed civilians 16+, 2) 2018-22 county data on the number of SE by incorporation status and gender, 3) 2015 national data on the fraction of the SE without employees by incorporation status, and 4) 2019 data on the fraction of the SE with no employees by gender. The potential value is likely an upper-estimate due to how industry mix affects the local self-employment rate. Whether a self-employed individual holds one or more jobs would also effect the potential, but this effect is unknown. Rural areas tend to have a higher fraction of individuals who are self-employed and the ability to pull them into employment may not be as high as in urban areas. Base population = civilian employed population 16+.
5.1 Annual data. Apprenticeships completed per year. On-going research.
5.2 Annual data. The number of internships completed each year. No state or county data is available for internships. The estimates provided here are based on the author's research on internships at one large Midwest university (2015-18 data). Internship locations were first correlated with county civilian labor force and RUCC data and then county estimates were made across the U.S.. A second estimate was made based on county-level data on the number of students enrolled in four-year colleges and graduate schools (2018-22) and various studies on the fraction of students who complete an internship. These values were then adjusted in some cases to address outliers (e.g. small population counties with universities). Both estimates were then scaled to match national estimates of the number of annual internships. These two different approaches were then averaged to get the results presented here. Base population = CLF 16+.
5.3 Youth programs include 4-H, Boy Scouts, Girl Scouts, and FIRST LEGO League participants. Values are the total number of individuals participating in a given year, which covers individuals in different years in school. Data on these programs is not available across the country, so estimates are made using Iowa data. The county participation estimate is based on the number of youth 5-17 in the county in 2018-2022 and the 2014-2018 Iowa participation rate data. The workforce potential assumes the standard deviation of the U.S. county participation rate is the same as the 2014-18 SD for Iowa. Base population = Population 5-17.